Generating Diverse Agricultural Data for Vision-Based Farming Applications
Published at: 2024 IEEE/CVF Conference on Computer Vision and Pattern Recognition Workshops (CVPRW)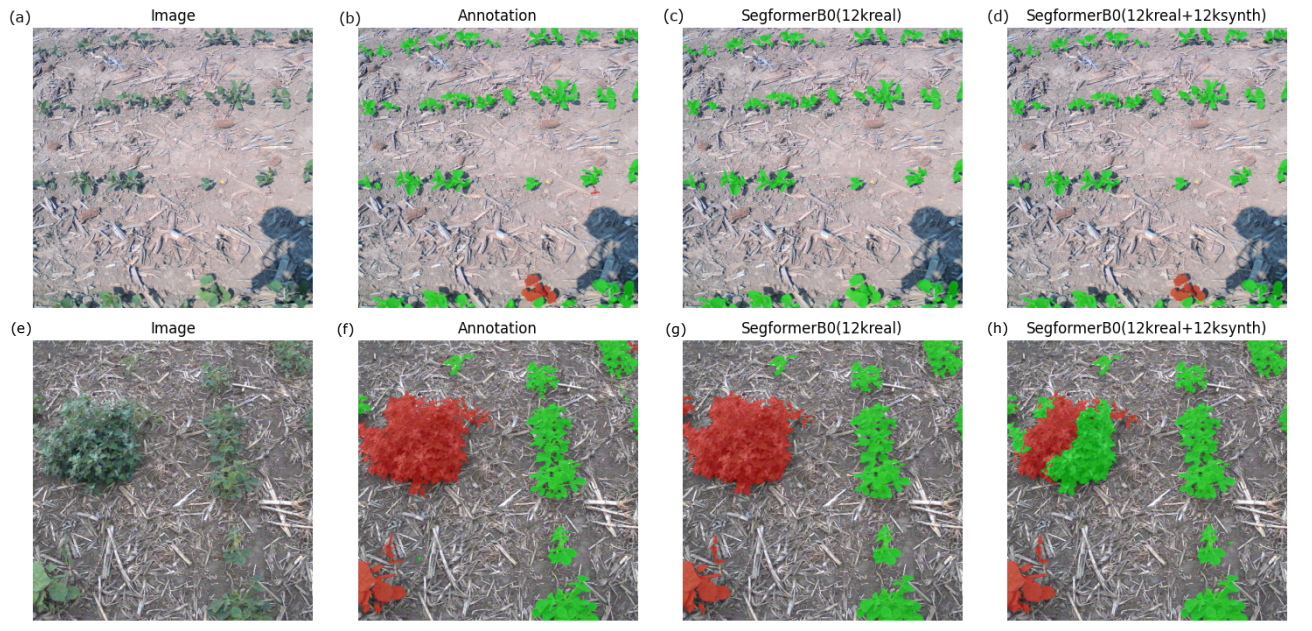
Abstract
We present a specialized procedural model for generating synthetic agricultural scenes, focusing on soybean crops, along with various weeds. This model is capable of simulating distinct growth stages of these plants, diverse soil conditions, and randomized field arrangements under varying lighting conditions. The integration of real-world textures and environmental factors into the procedural generation process enhances the photorealism and applicability of the synthetic data. Our dataset includes 12,000 images with semantic labels, offering a comprehensive resource for computer vision tasks in precision agriculture, such as semantic segmentation for autonomous weed control. We validate our model's effectiveness by comparing the synthetic data against real agricultural images, demonstrating its potential to significantly augment training data for machine learning models in agriculture. This approach not only provides a cost-effective solution for generating high-quality, diverse data but also addresses specific needs in agricultural vision tasks that are not fully covered by general-purpose models.
BibTeX
@inproceedings{AgriData2024, title={Generating Diverse Agricultural Data for Vision-Based Farming Applications}, author={Mikolaj Cieslak and Umabharathi Govindarajan and Alejandro Garcia and Anuradha Chandrashekar and Torsten Hadrich and Aleksander Mendoza-Drosik and Dominik Michels and Soren Pirk and Chia-Chun Fu and Wojtek Palubicki}, booktitle={2024 IEEE/CVF Conference on Computer Vision and Pattern Recognition}, year={2024}, keywords={Virtual crops, data augmentation, transfer learning, semantic segmentation}, doi={} }